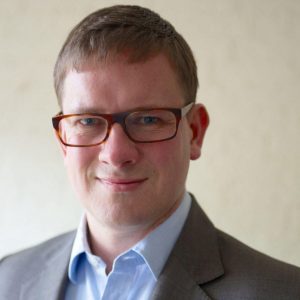
Andrew’s education is in Economics & Mathematics. After working in management consultancy and in senior HR positions he founded OrganizationView in 2010. He is a regular conference speaker and chair, teaches HR analytics in businesses and at the university. He is a member of the CIPD’s advisory group on HR analytics.
What are the key aspects of employee performance that are critical to the success of HR Analytics?
For analyses, the most important part is to identify clearly what it is that you’re trying to optimize. Often this is not intuitive.
Take for example the frequent study of looking at attrition. The original question might be that we want to reduce attrition but this is rarely the case. What is more usual is that businesses want to reduce the cost of attrition. Employees aren’t equal. Most businesses will do more to keep high-performers and might be glad a poor performer resigns. Certain skill sets might be critical to the business or hard to recruit. Salespeople might take their customers so you need to factor in lost revenue. It might take 2 days to bring someone in the call center up to speed but 9 months before an executive is working effectively.
If you just try to reduce overall attrition you’ll probably find your analyses will ignore all of these challenges.
How can HR Analytics enhance employee performance?
HR Analytics typically works by splitting your organization into different groups and identifying a probability for each group of meeting some objective. So it might be to identify the conditions that increase the likelihood that someone resigns in the next time period. We might say that people under the age of 30, without a university education, that received less than a 5% salary increase and live more than 45 minutes from the office have a 20% higher chance of resigning. People meeting these conditions will form a different group.
What do CEOs/CHROs look for in employee performance analytics?
In our experience, the analyses that have the most impact are those that address key business challenges. So it might be exploring what are the factors that increase the likelihood that someone in sales is a high performer or whether it is more cost effective to hire graduates or more experienced staff. These are typically analyses that you can put a sensible financial value against. Making it more tangible, and being able to present a range of scenarios with the cost/benefit of each option is important.
What is missing in terms of employee performance data that could make HR Analytics even more meaningful?
The big missing data is usually perception data. Companies will have data to answer ‘who’, ‘when’, ‘what’ but will rarely have information that answers ‘why’. To take meaningful actions you need to understand why something is happening.
In our experience when you ask employees about what is causing something, or what they need to perform better, you get a much better signal than just using demographic or behavioral data. In the past HR might have asked these questions but not linked it to the data about behaviors and demographics. The first wave of HR analytics used the behaviors and demographic data but not the perceptions.
When we do link the two together we usually see that 4 of the top 10 drivers might be related to this feedback data. They’re also likely to be the factors that are most easy to action.
It was this need to acquire perception data for models, usually as an open text, that drove us to build Workometry. (https://www.workometry.com) It’s being used by many of the worlds smartest HR analytics teams and management consultancies.
Can HR Analytics play a prescriptive role in helping employee finetune performance real-time?
In some instances. To do this the limiting factor is not the HR Analytics but the need to have real-time performance data to build the model against. Very few areas of the business generate this information.
There are analyses that make sense to move into production. For example, we’re helping one large firm understand what employees and their managers agree in their development needs and to make recommendations of training programs or other development actions. You can start relatively simple here and increase the sophistication. At the moment because this data is in multiple systems businesses will probably have to build this themselves. Over the next few years, we’ll start to see more and more companies integrate these predictions into their systems.